Despite the emergence of innovative architectures claiming improved capabilities in modeling human-level creativity, state-of-the-art generative music systems still struggle with creating musical content that follows technical rules and expectations.
The conventional subjective evaluation method for generative models can introduce bias and also lacks transparency, rigor, and reproducibility, emphasizing the need for more objective metrics. However, existing approaches to objective evaluation have either relied on overly-broad criteria that do not capture higher-level music theoretic properties nor perceptual properties, or are narrowly tailored to the design of a specific model, limiting their generalizability.
We propose a reproducible and highly interpretable approach for evaluating the output of symbolic music generation models, employing musicologically-informed objective metrics. We evaluate established and cutting-edge generative models by comparing the models’ training data against the generated results through systematic computational musicological analysis. By examining the model under these metrics, we can formatively assess if the model behaves as expected and identify areas for improvement.
Overall, our study provides a means to formulate detailed evaluation with respect to human- interpretable characteristics, and offers a more comprehensive and reliable evaluation procedure.
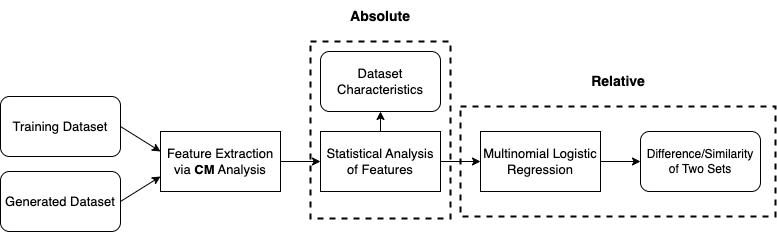